
Daniel J.
Mankowitz
Co-Founder & CTO @ Ethos
www.askethos.com
Ex. Staff Research Scientist @ Google Deepmind | AlphaDev
Email: daniel (dot) mankowitz (at) gmail (dot) com
About Me
I am currently a co-founder & CTO of Ethos. Previously, I was a Staff Research Scientist at Google Deepmind.
My work involved building and improving important real-world applications and products using Reinforcement Learning and Large Language Models (LLMs). This includes a focus on Reinforcement Learning from Human Feedback (RLHF) for Language Models such as Bard and Gemini.
I have published works in Nature and Science which include:
Faster sorting algorithms discovered by deep reinforcement learning - Nature (2023)
Featured as one of Google's Ground-breaking AI Advances of 2023
Competition-level code generation with AlphaCode - Science (2022)
Featured as one of Science's break-throughs of the year
Press

Applications I have worked on include:
-
Code optimization
-
Code generation
-
Chip Design
-
Video Compression
-
Recommender Systems
-
Controlling physical systems such as Heating Ventilation and Air-Conditioning (HVAC)
-
Plasma Magnetic Control
A summary of the impact of some of this work is in the Google Deepmind blog post below:
Featured Talks
Optimizing the Computing Stack using AI from the Software to the underlying Hardware - CogX 2023
AI Writes Code that runs 70% faster than ours - The Naked Scientist 2023
AlphaDev: Faster Sorting Algorithms discovered using deep RL - Imperial College London - 2023
Skills

Work History

Education
In 2018, I completed my PhD in Hierarchical Reinforcement Learning under the supervision of Professor Shie Mannor, at the Technion Israel Institute of Technology. I am a recipient of the Google PhD Fellowship.
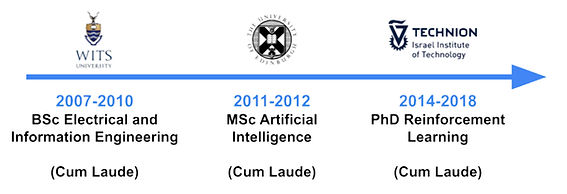
Previous Ventures

Jan 2014- June 2015
Press: Times of Israel, Globes
We at Fitterli are unleashing the power of the 3D depth camera. We are developing the first body digitization application that will allow you to digitize yourself with your tablet or laptop with a 3D-enabled camera. This app will automatically extract your exact body measurements and allow you to use a whole host of services which include tracking your body while following a diet or fitness regime, order bespoke clothes from tailors online, 3D virtual changerooms and much more!
Featured Papers
Press: Techcrunch, Nature, VentureBeat, NewScientist, Wired, Wall Street Journal, MIT Technology Press
Fundamental algorithms such as sorting or hashing are used trillions of times on any given day. As demand for computation grows, it has become critical for these algorithms to be as performant as possible. Whereas remarkable progress has been achieved in the past, making further improvements on the efficiency of these routines has proved challenging for both human scientists and computational approaches. Here we show how artificial intelligence can go beyond the current state of the art by discovering hitherto unknown routines. To realize this, we formulated the task of finding a better sorting routine as a single-player game. We then trained a new deep reinforcement learning agent, AlphaDev, to play this game. AlphaDev discovered small sorting algorithms from scratch that outperformed previously known human benchmarks. These algorithms have been integrated into the LLVM standard C++ sort library. This change to this part of the sort library represents the replacement of a component with an algorithm that has been automatically discovered using reinforcement learning. We also present results in extra domains, showcasing the generality of the approach.
"Software Engineered
Game-playing AI speeds up
sorting in computer code" - Nature Cover Feature

Paper | Blog | New York Times
This report introduces a new family of multimodal models, Gemini, that exhibit remarkable capabilities across image, audio, video, and text understanding. The Gemini family consists of Ultra, Pro, and Nano sizes, suitable for applications ranging from complex reasoning tasks to on-device memory-constrained use-cases. Evaluation on a broad range of benchmarks shows that our most-capable Gemini Ultra model advances the state of the art in 30 of 32 of these benchmarks — notably being the first model to achieve human-expert performance on the well-studied exam benchmark MMLU, and improving the state of the art in every one of the 20 multimodal benchmarks we examined. We believe that the new capabilities of the Gemini family in cross-modal reasoning and language understanding will enable a wide variety of use cases. We discuss our approach toward post-training and deploying Gemini models responsibly to users through services including Gemini, Gemini Advanced, Google AI Studio, and Cloud Vertex AI.

Video streaming usage has seen a significant rise as entertainment, education, and business increasingly rely on online video. Optimizing video compression has the potential to increase access and quality of content to users, and reduce energy use and costs overall. In this paper, we present an application of the MuZero algorithm to the challenge of video compression. Specifically, we target the problem of learning a rate control policy to select the quantization parameters (QP) in the encoding process of libvpx, an open source VP9 video compression library widely used by popular video-on-demand (VOD) services. We treat this as a sequential decision making problem to maximize the video quality with an episodic constraint imposed by the target bitrate. Notably, we introduce a novel self-competition based reward mechanism to solve constrained RL with variable constraint satisfaction difficulty, which is challenging for existing constrained RL methods. We demonstrate that the MuZero-based rate control achieves an average 6.28% reduction in size of the compressed videos for the same delivered video quality level (measured as PSNR BD-rate) compared to libvpx's two-pass VBR rate control policy, while having better constraint satisfaction behavior.
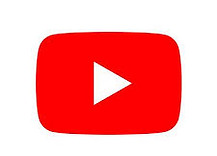
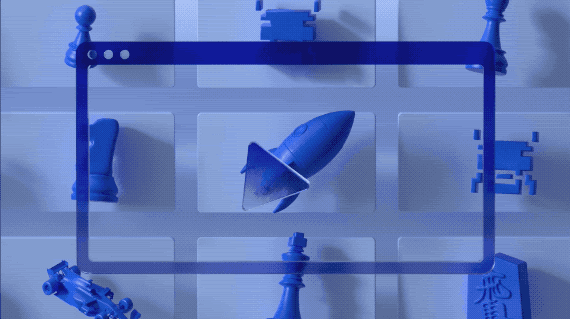
Competition-Level Code Generation with AlphaCode (Science 2022)
Featured in top 10 break-throughs of the year by Science
Press: Science, Techcrunch, VentureBeat, The Times
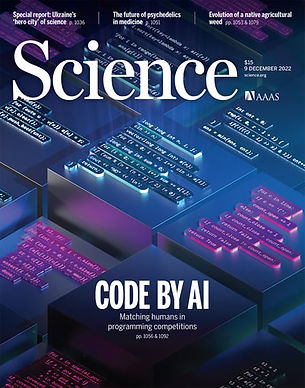

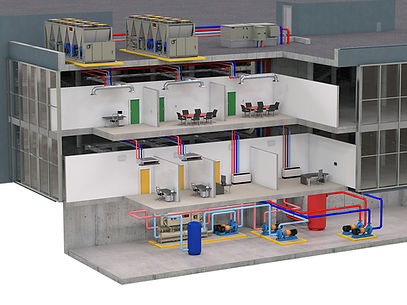
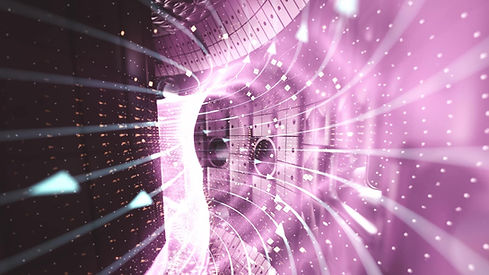
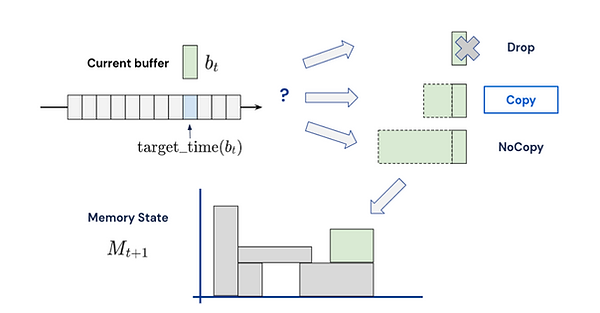
Reinforcement Learning (RL) has proven to be effective in solving numerous complex problems ranging from Go, StarCraft and Minecraft to robot locomotion and chip design. In each of these cases, a simulator is available or the real environment is quick and inexpensive to access. Yet, there are still considerable challenges to deploying RL to real-world products and systems....
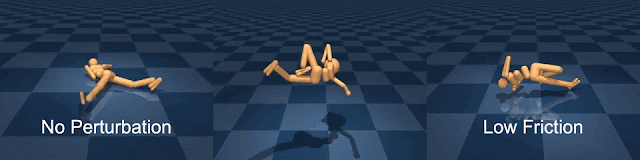
Selected Publications
Paper | ICML 2019 RL4RealLife Workshop (2019) Best paper award
We present a set of nine unique challenges that must be addressed to productionize RL to real world problems....
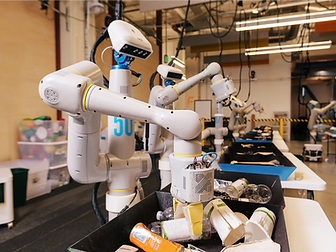
Paper | Springer Special Issue on RL for Real Life (2021)
Our proposed challenges are implemented in a suite of continuous control environments called realworldrl-suite which we propose an as an open-source benchmark...
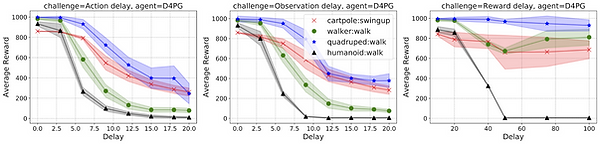
Paper | ICLR (2019)
In this work we present a novel multi-timescale approach for constrained policy optimization, called `Reward Constrained Policy Optimization' (RCPO), which uses an alternative penalty signal to guide the policy towards a constraint satisfying one. We prove the convergence of our approach and provide empirical evidence of its ability to train constraint satisfying policies.
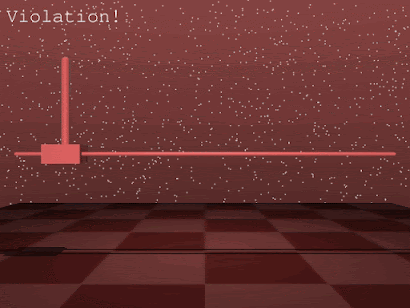
Paper | AAAI 2017
We propose a lifelong learning system that has the ability to reuse and transfer knowledge from one task to another while efficiently retaining the previously learned knowledge-base ... The H-DRLN exhibits superior performance and lower learning sample complexity compared to the regular Deep Q Network (Mnih et. al. 2015) in sub-domains of Minecraft.

Paper | ICLR 2020
We provide a framework for incorporating robustness -- to perturbations in the transition dynamics which we refer to as model misspecification -- into continuous control Reinforcement Learning (RL) algorithms....

Paper | RLDM 2019
We propose a novel agent architecture called Unicorn, which demonstrates strong continual learning and outperforms several baseline agents on the proposed domain. The agent achieves this by jointly representing and learning multiple policies efficiently, using a parallel off-policy learning setup.
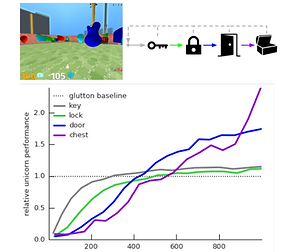